The remarkable advancements in AI have given rise to intelligent systems capable of autonomous decision-making and problem-solving. As AI becomes more pervasive, it is becoming increasingly important to ensure that AI systems are responsible and auditable. While Responsible AI focuses on ethical considerations and ensuring that AI systems make fair and unbiased decisions, auditability emphasizes the need for fair, equitable, and transparent AI models for organizations to build trust with stakeholders and end-users.
In this blog post, we will discuss the concept of auditable AI and explore the essential steps required to make your AI systems auditable, enhance trust, mitigate risks, and unlock the full potential of auditable AI.
Is your AI Auditable?
Auditable AI is a set of principles and practices that can be used to ensure that AI systems are transparent, accountable, and reliable. Auditable AI provides insights into how AI arrives at its decisions, identifies biases, and ensures compliance with ethical guidelines. Auditable AI holds significant importance in today’s technology-driven world for several reasons.
Documentation: Document all aspects, including the data used to train the model, the algorithms used, and the decision-making process.
Traceability: The AI system should be able to trace, explain and audit its decisions to the stakeholders.
Transparency: The AI system should be transparent to users so that they can understand how it works and make informed decisions.
Accountability: It allows for the mitigation of risks in AI systems, ensuring that decisions align with established objectives and ethical considerations.
Responsible AI or Auditable AI?
Both responsible AI and auditable AI are important approaches to ensuring the responsible development and use of AI. RAI is more comprehensive, but it can be more difficult to implement. AAI is more technical, but it can be more effective at ensuring the fairness and transparency of AI systems. By embracing both responsible AI and auditable AI, organizations can reap numerous benefits.
Feature | Responsible AI | Auditable AI |
Focuses mainly on | Ethical Principles and Fairness in AI Development and Deployment | Transparency and Accountability in the AI decision-making process |
The aim is to | Mitigate biases and ensure non-discriminatory outcomes | Provide visibility into factors influencing AI outcomes, algorithms, and data inputs |
Method of implementation | Includes principles such as fairness, bias avoidance, transparency, and accountability | Includes techniques such as data logging, algorithm auditing, and decision tracing |
Best practices include | Implement principles and practices throughout the AI lifecycle. Monitor and review AI systems on an ongoing basis | Log data, audit algorithms, and trace decisions. Use visualization tools to make AI systems more understandable |
Challenges | RAI can be more difficult and expensive to implement | AAI is more complex and requires specialized skills |
Steps to Build an Auditable AI
Organizations must strive for auditable AI systems to build trust and transparency. Let’s look at the essential steps to achieving auditable artificial intelligence, going beyond mere responsibility.
Step 1: Establish a governance framework
Assemble a team, including AI experts, data scientists, legal professionals, and representatives, to develop policies and procedures. These guidelines should encompass data labeling, version control, and proper documentation to manage AI risks.
Additionally, organizations should consider implementing data anonymization techniques to protect individual privacy and comply with relevant data protection regulations.
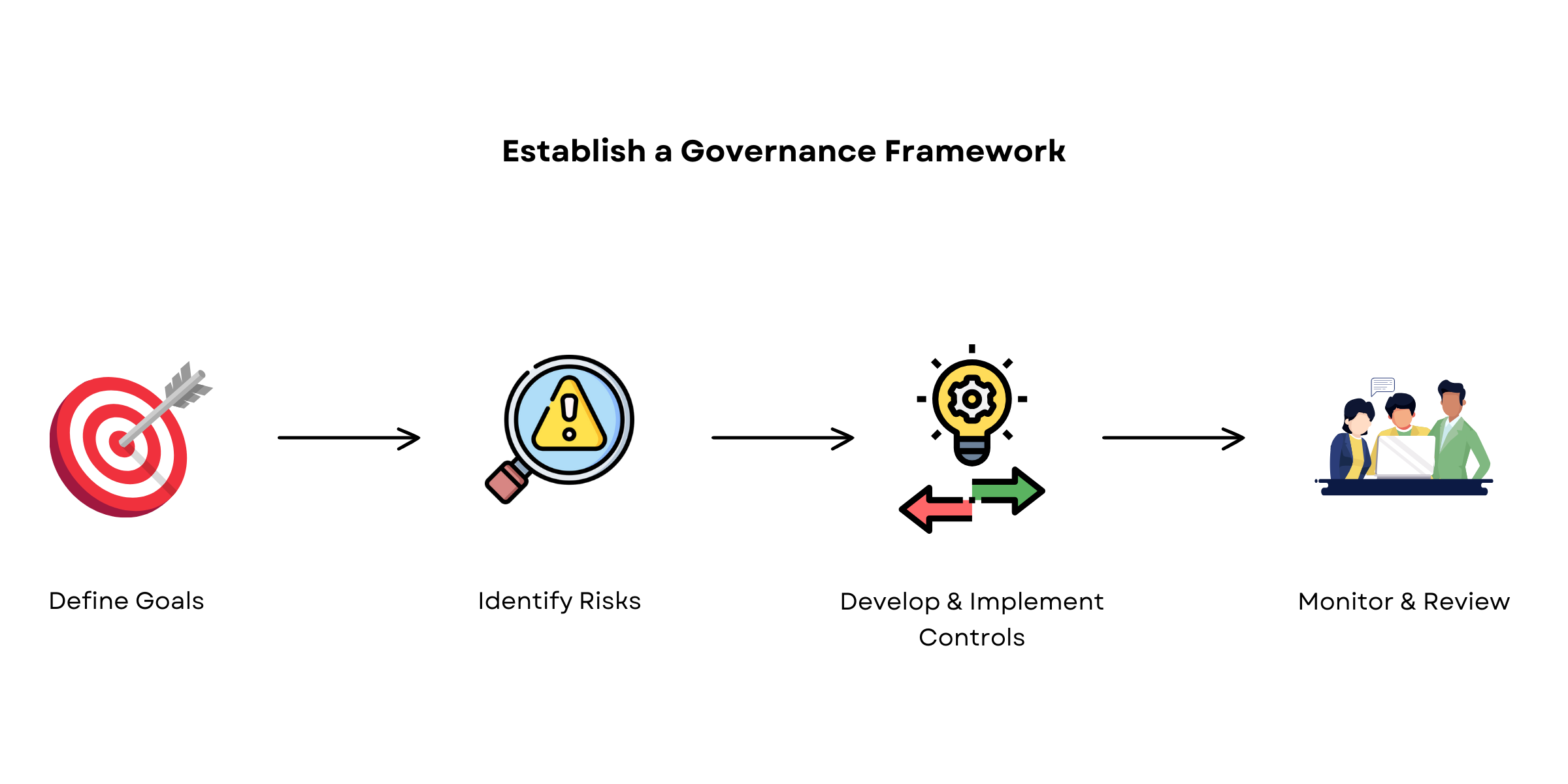
Step 2: Establish Objectives and Metrics
Objectives provide a roadmap, helping define the desired outcomes and ensuring that auditable AI aligns with organizational values, and enabling stakeholders to understand the purpose and benefits of auditable AI, fostering support and buy-in across the organization.
Metrics play a pivotal role in assessing the effectiveness and performance of auditable AI systems. When defining metrics, consider process-oriented metrics for transparency, and explainability and outcome-oriented measurements for mitigating biases, compliance adherence, and the accuracy and fairness of AI-driven decisions.
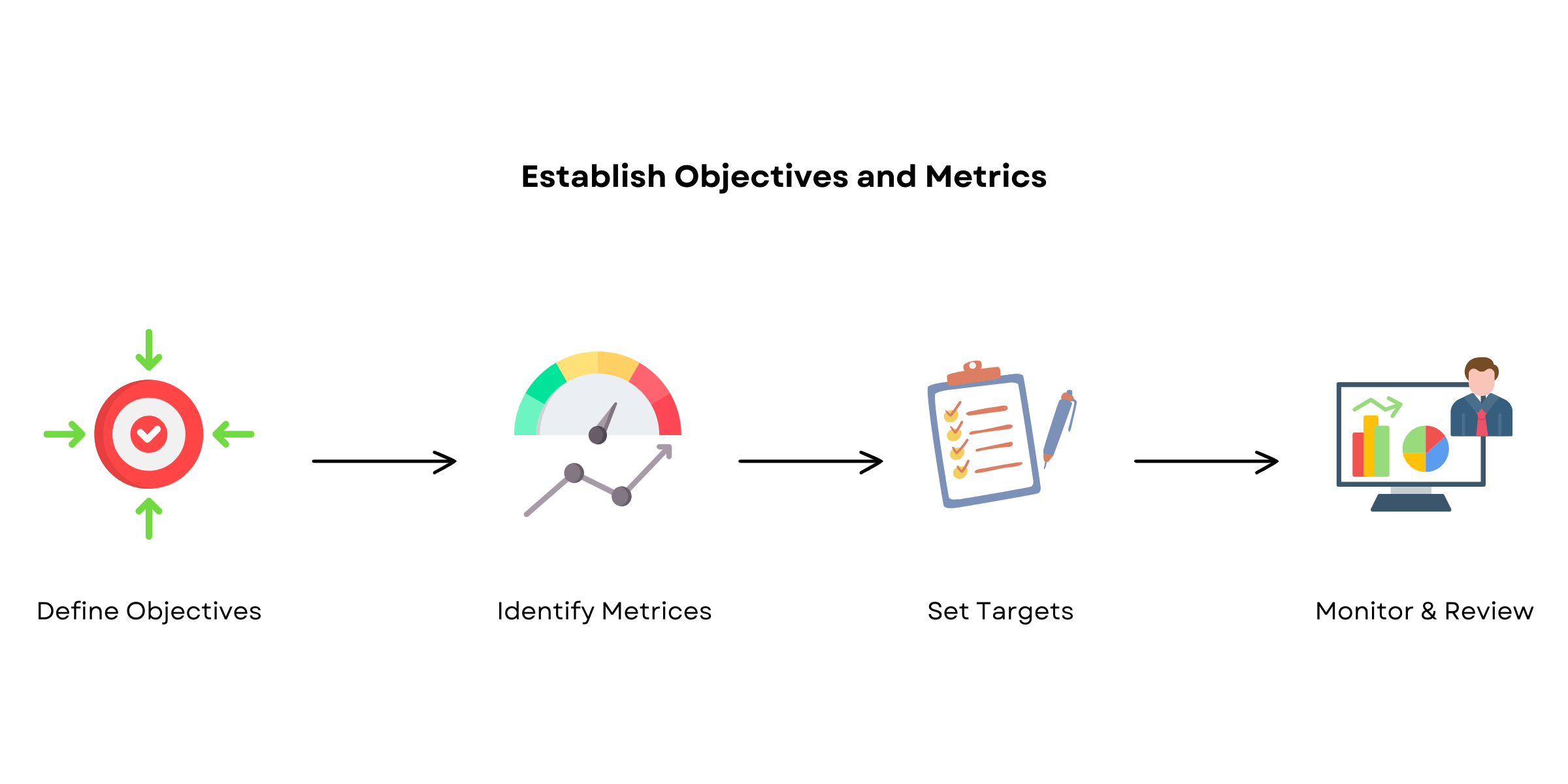
Step 3: Incorporate Explainable AI Techniques
Explainable AI techniques are the foundation for achieving auditable AI by explaining the underlying logic, factors, and features influencing AI outcomes. Through techniques such as rule-based models, interpretable machine learning, local explanations, and visualizations, organizations can enhance the explainability of AI decisions.
By understanding stakeholder needs, enhancing data governance, collaborating with experts, and documenting the AI development process, organizations can effectively implement explainable AI techniques and establish auditable AI practices.
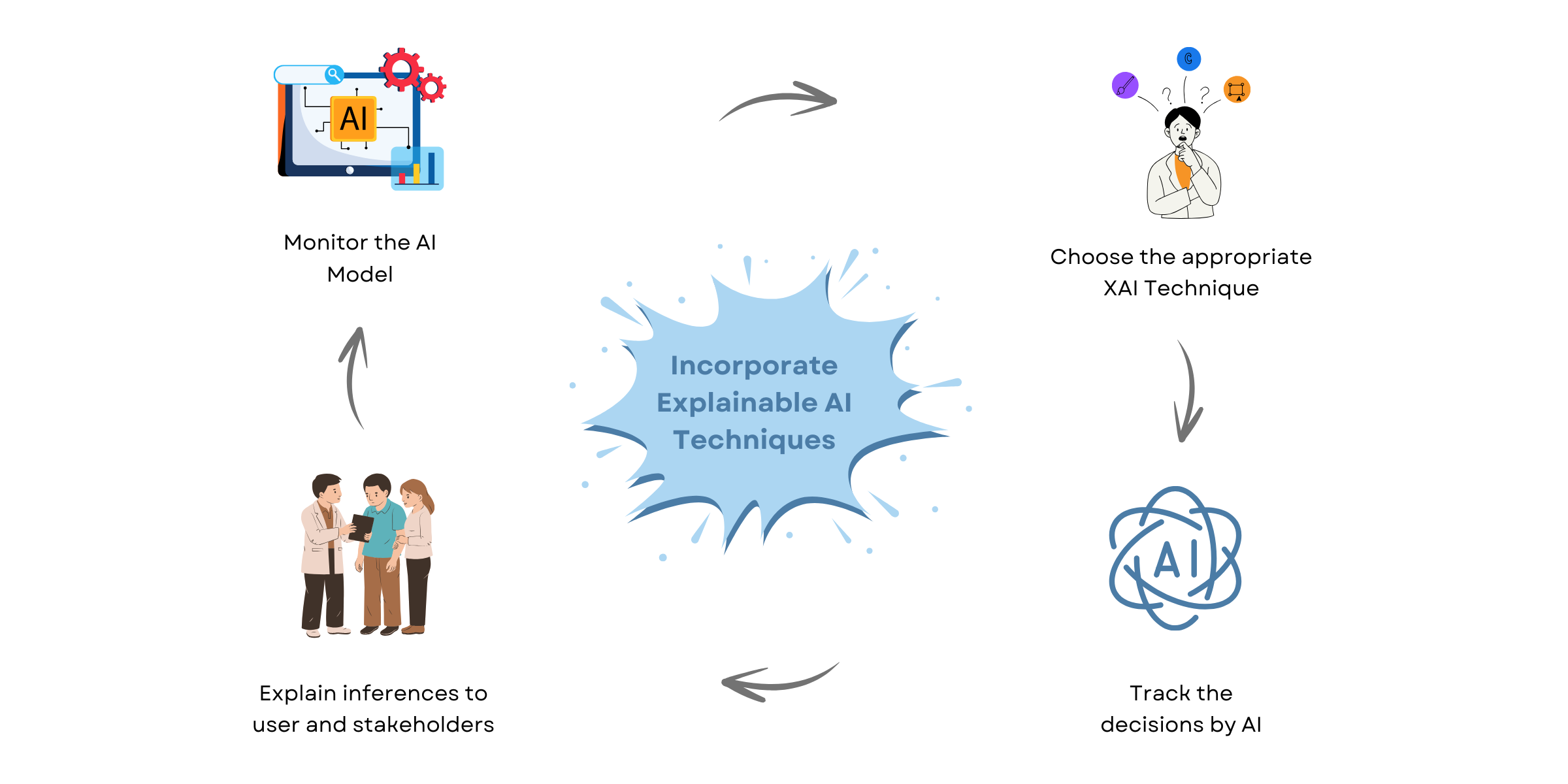
Step 4: Deploy AI Models Responsibly
Responsibly deploying AI models not only enhances transparency but also ensures accountability and enables effective auditing. By considering factors such as data governance, bias detection and mitigation, explainability, and ongoing monitoring, organizations can foster transparency and accountability throughout the AI lifecycle.
Through cross-functional collaboration, robust documentation, and continuous evaluation, organizations can effectively deploy and build trustworthy AI systems that promote transparency, mitigate biases, and instill confidence among stakeholders.
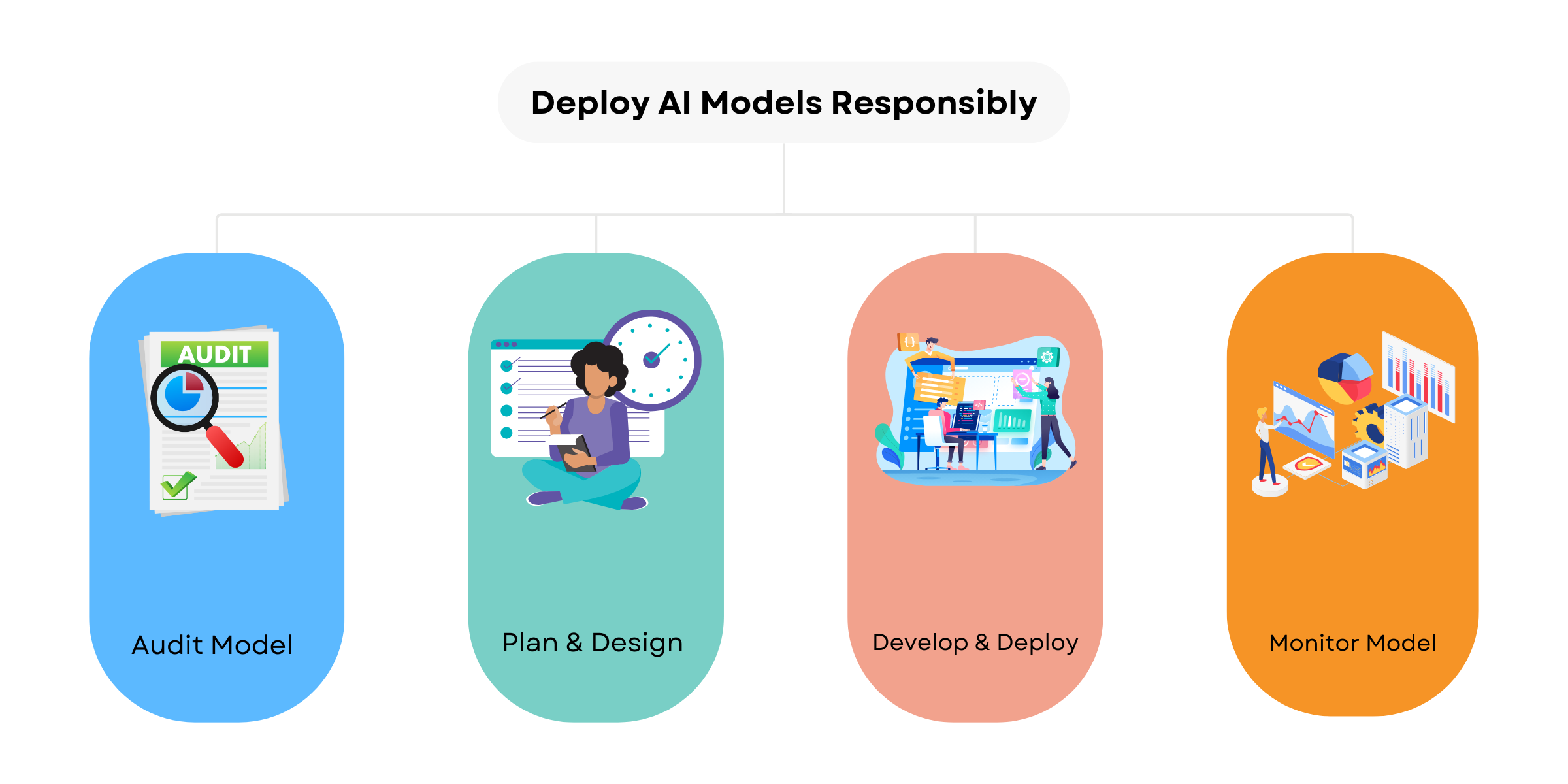
Step 5: Monitor Models and Establish Feedback Loops
By monitoring models, organizations can identify and address issues such as biases, data drift, and concept drift that may affect the fairness, accuracy, and compliance of AI outputs. Monitoring also enables organizations to track the behavior of AI models in real-world scenarios, detect anomalies, and ensure that the models align with the intended objectives and ethical guidelines.
Feedback loops serve as a crucial mechanism for gathering insights and incorporating stakeholder feedback into AI model development and deployment. Effective feedback loops enable organizations to engage stakeholders, gather user feedback, assess ethical implications, and drive continuous learning and improvement.
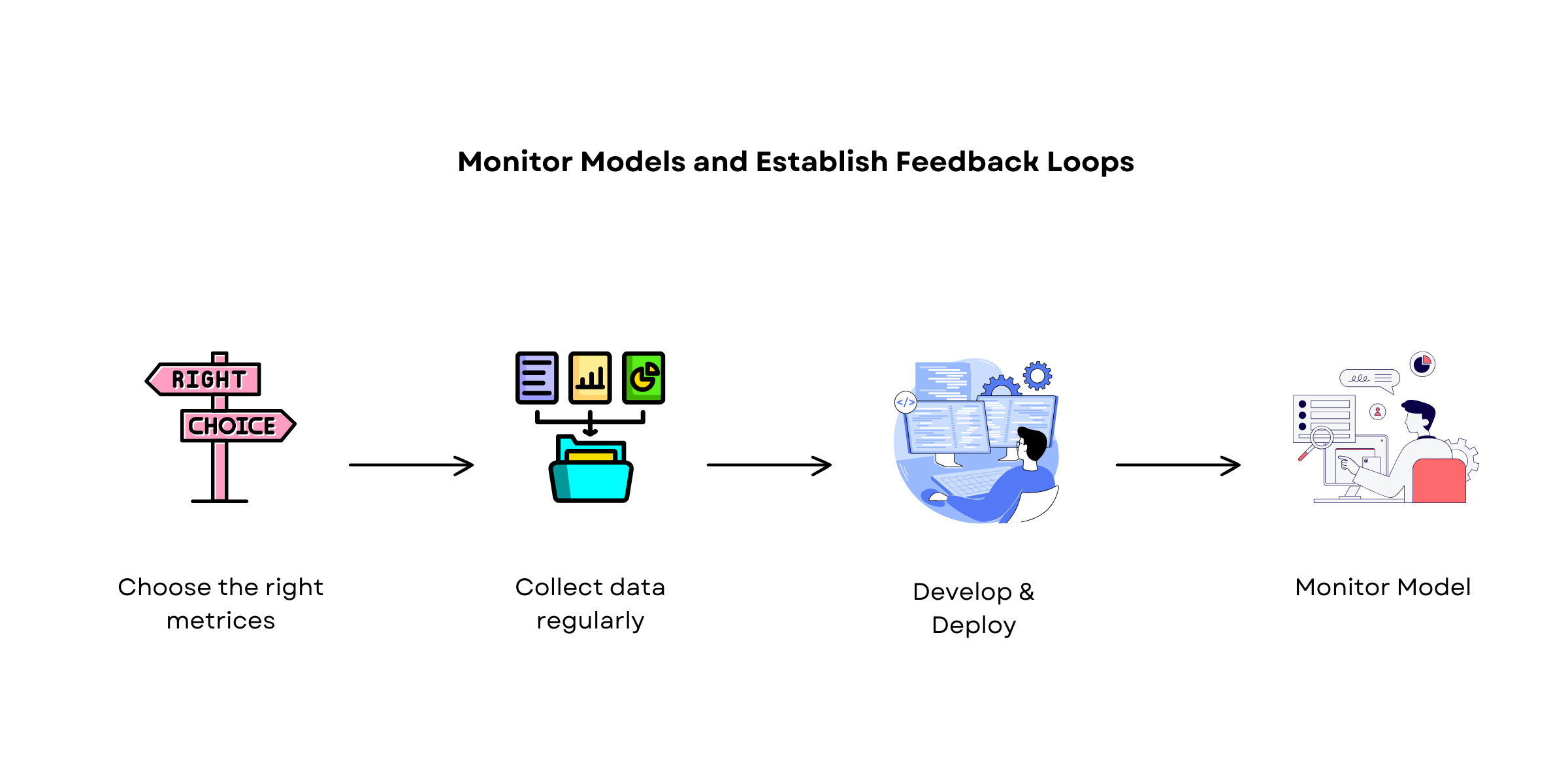
Step 6: Document the Model Processes
Documentation throughout the AI lifecycle provides a comprehensive record of the model journey, facilitating audits, evaluations, and regulatory compliance. Documented processes enable stakeholders, regulators, and internal teams, to gain insights into the underlying methodologies, data sources, and decision-making processes employed by the AI models.
By documenting the methodology, data collection, preprocessing, model architecture, hyperparameters, training, evaluation, and ethical considerations, organizations can enhance transparency, traceability, and accountability throughout the AI lifecycle.
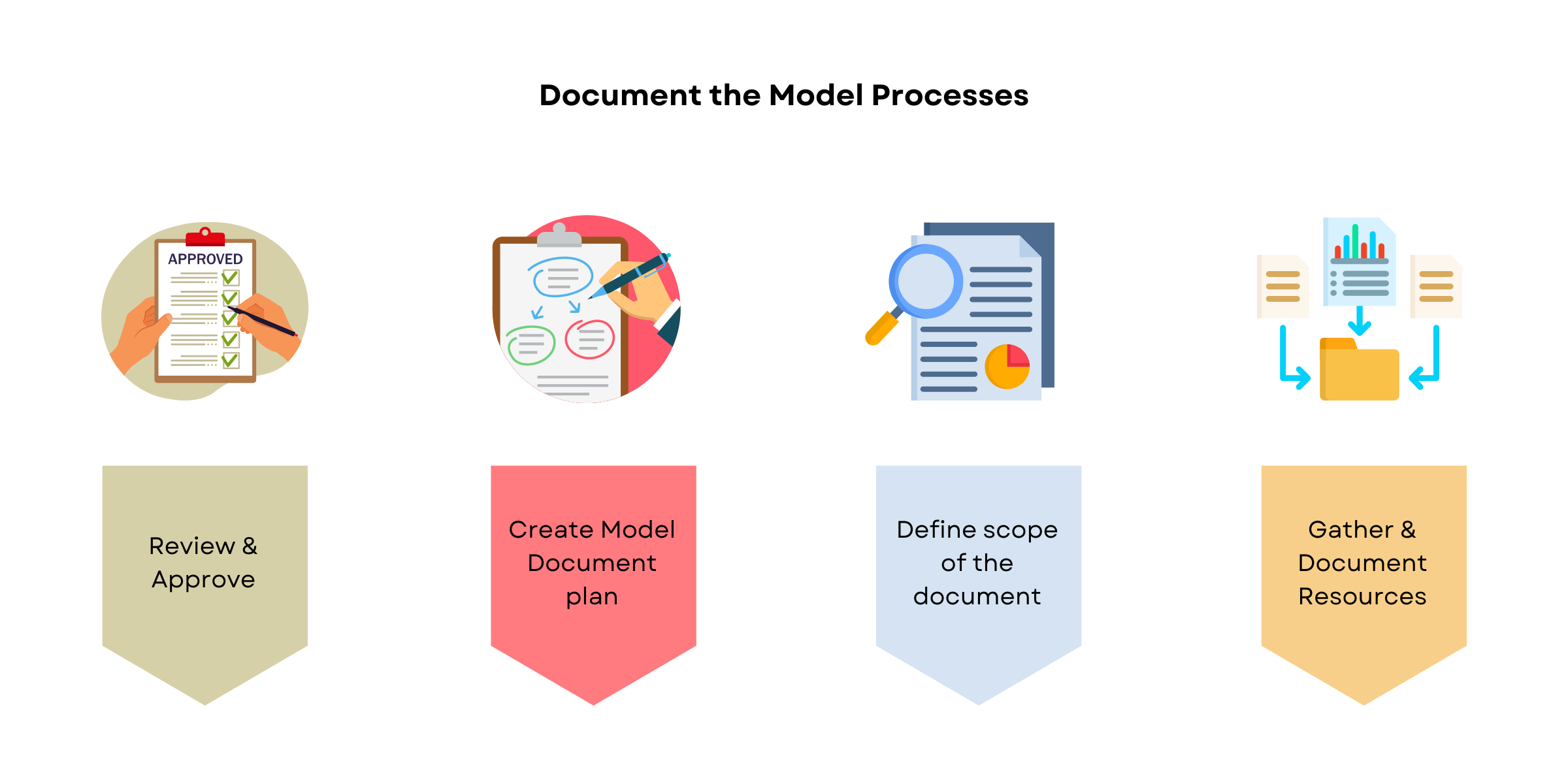
Step 7: Audit AI Systems Regularly
Audits provide a comprehensive assessment of AI systems, ensuring compliance with ethical guidelines, regulatory requirements, and organizational policies. By conducting regular audits, organizations can identify and rectify any shortcomings, instill trust among stakeholders, and enhance the overall quality and reliability of AI systems.
By auditing AI systems regularly, organizations can increase transparency by providing users and stakeholders with information about how the systems work and can improve accountability and mitigate biases by providing a way to track and measure the performance of the systems.
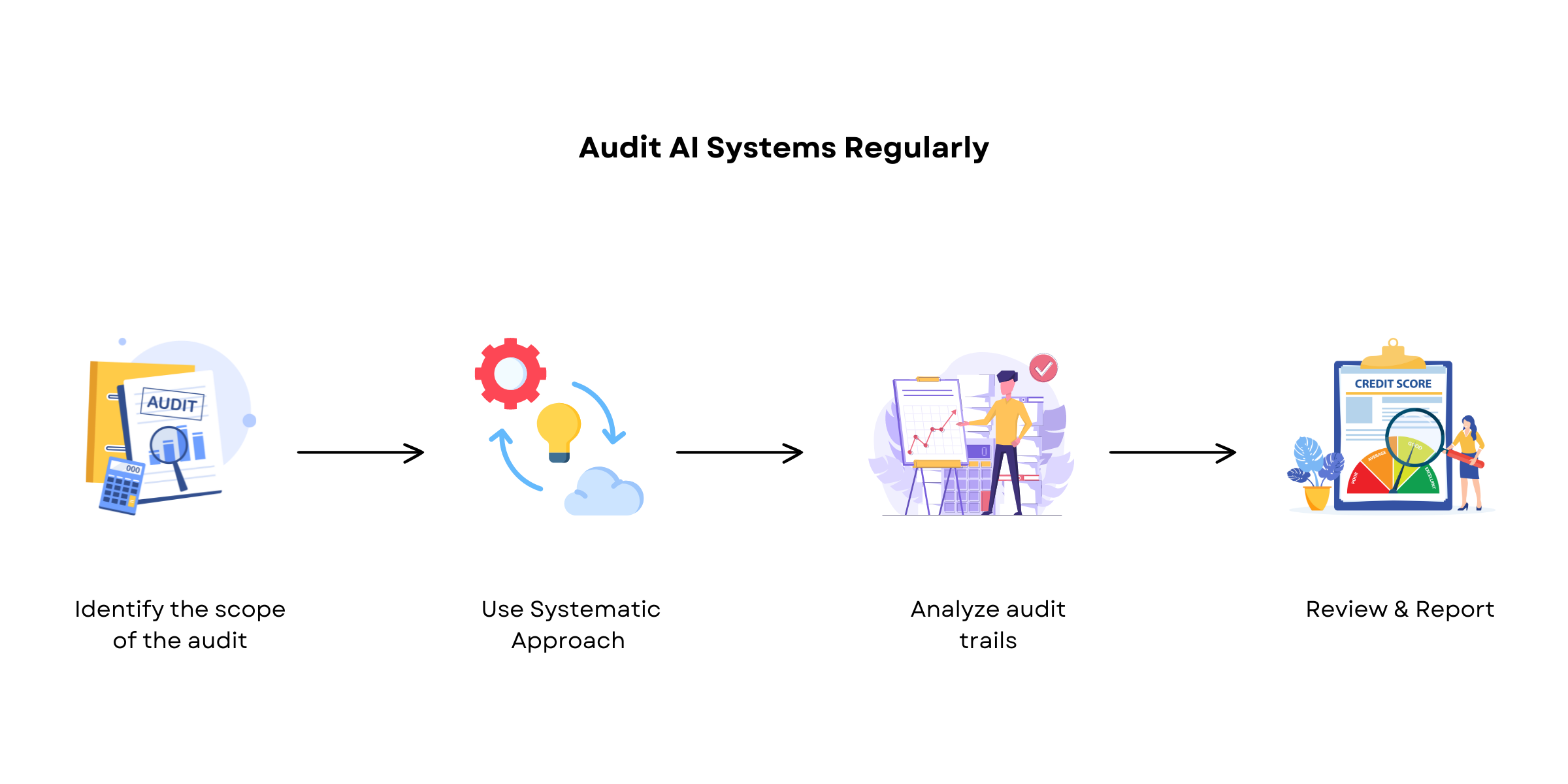
To Wrap Up
Building auditable AI systems is essential for fostering trust, mitigating risks, and deciphering the full potential of AI. By establishing a governance framework, defining objectives and metrics, incorporating explainable AI techniques, responsibly deploying AI models, monitoring and establishing feedback loops, documenting model processes, and conducting regular audits, organizations can cultivate transparent, accountable, and reliable AI systems that benefit businesses.
Leave A Comment